What is AI Risk Management?
AI risk management refers to the process of identifying, assessing, and mitigating risks associated with the development, deployment, and use of artificial intelligence systems. This process is crucial for ensuring that AI technologies are used safely, ethically, and effectively. Key components of AI risk management include:
- Risk Identification: Recognizing potential hazards and adverse outcomes that may arise from the use of AI, such as biases in decision-making, privacy breaches, or unintended consequences of AI actions.
- Risk Assessment: Evaluating the likelihood and impact of identified risks. This involves analyzing how AI systems make decisions, the data they use, and the contexts in which they operate to determine the potential for harm or error.
- Risk Mitigation Strategies: Developing and implementing measures to reduce or eliminate identified risks. This can include designing AI systems with ethical considerations in mind, ensuring transparency and explainability in AI decision-making, and incorporating fail-safes.
- Monitoring and Review: Continuously monitoring AI systems post-deployment to detect any emerging risks or unforeseen issues. This process involves collecting and analyzing data on the system's performance and impact.
- Compliance with Laws and Regulations: Ensuring that AI systems comply with existing laws, standards, and ethical guidelines. This includes data protection regulations, non-discrimination laws, and industry-specific regulations.
- Stakeholder Engagement: Involving a diverse range of stakeholders, including users, ethicists, legal experts, and the public, in discussions about AI deployment and its societal impacts.
- Education and Training: Educating developers, users, and decision-makers about the capabilities, limitations, and risks of AI systems to promote informed and responsible use.
- Contingency Planning: Preparing for potential failures or negative outcomes of AI systems, including having plans for human intervention and system shutdowns if necessary.
Effective AI risk management requires a multi-disciplinary approach, combining technical expertise with insights from social sciences, ethics, and legal perspectives. As AI technologies continue to evolve, so will the strategies and practices for managing their associated risks.
What is AI Risk Management Framework?
An AI risk management framework is a structured approach to identifying, assessing, and mitigating risks associated with AI systems. On Jan. 26, 2023, National Institute of Standards and Technology (NIST) released the AI Risk Management Framework. This framework is designed to ensure the safe, ethical, and effective use of AI technologies, particularly in environments where their decisions can have significant consequences.
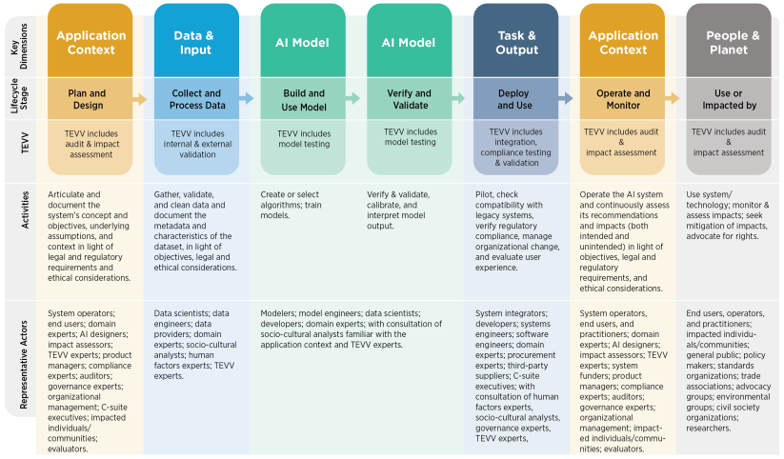
AI actors across AI lifecycle stages defined in NIST.AI.100-1.
Key elements of an AI risk management framework typically include:
- Governance and Leadership: Establishing clear roles and responsibilities for overseeing AI risk management. This involves setting up governance structures and leadership teams to guide and enforce AI risk strategies.
- Policy and Standards Development: Creating policies and standards that define acceptable use of AI, ethical guidelines, compliance requirements, and risk management procedures.
- Risk Assessment: Systematically identifying and evaluating potential risks that AI systems might pose. This involves analyzing the probability and impact of risks such as bias, error, security vulnerabilities, and ethical concerns.
- Control Implementation: Developing and implementing controls to mitigate identified risks. This includes technical measures like robust AI testing, data integrity checks, and security protocols, as well as organizational measures like training and awareness programs.
- Monitoring and Reporting: Continuously monitoring AI systems to detect any emerging risks or issues and reporting on risk management activities to stakeholders. This includes setting up mechanisms for regular review and audits of AI systems.
- Incident Response and Recovery: Establishing procedures for responding to AI-related incidents or failures, including mechanisms for containment, investigation, and learning from incidents.
- Continuous Improvement: Regularly reviewing and updating the risk management framework to reflect new insights, technological advancements, and evolving regulatory landscapes.
- Stakeholder Engagement: Involving a wide range of stakeholders, including AI developers, users, ethicists, and regulators, in the risk management process to ensure a comprehensive understanding of risks and their potential impacts.
A well-designed AI risk management framework helps organizations balance the benefits of AI with the need to manage potential risks effectively. It is particularly important in sectors where AI decisions can have significant ethical, legal, or safety implications, such as healthcare, finance, and autonomous vehicles.